Beginner's Guide to Machine Learning: Demystifying the World of AI
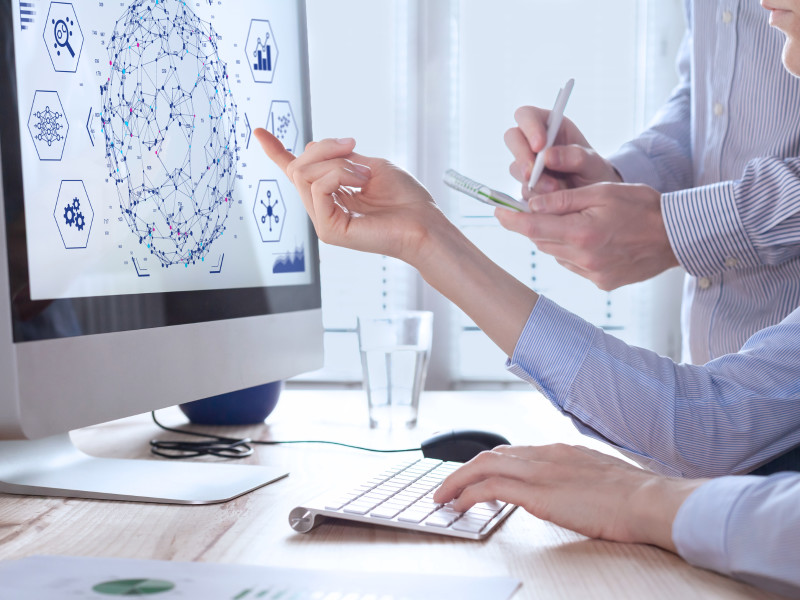
In this fast-paced and constantly evolving era, where technology is integrated into virtually every aspect of our lives, having a basic understanding of the fundamentals of machine learning is not just a privilege but essential.
Whether you are a curious enthusiast, a tech-savvy professional, or just someone who wants to keep up with the latest trends, this beginner's guide to machine learning is designed to give you a comprehensive and straightforward overview of one of the most exciting fields in modern computer science.
Understanding the Basics of Machine Learning
To understand the intricacies of machine learning, it's essential to grasp the basic principles underpinning this transformative technology. Machine learning involves using algorithms and statistical models to enable computer systems to automatically improve performance on a specific task by learning from data.
At the core of machine learning lies the crucial capability to detect data patterns. This involves providing an extensive set of data to a machine learning model, which can then detect patterns and trends that may not be easily distinguishable to humans. These patterns can then be leveraged to make predictions or facilitate decision-making processes.
There are three main types of machine learning—supervised learning, unsupervised learning, and reinforcement learning.
1. Supervised Learning
In supervised learning, labeled data is provided to the model during training. Labeled data refers to input data with the correct corresponding output or labeling. Tasks such as image classification and speech recognition commonly use this learning method. This method uses existing labeled data to identify patterns that can be applied to new, unlabeled data, thus teaching the model.
2. Unsupervised Learning
Unsupervised learning differs from supervised learning in presenting an unlabeled dataset to the machine learning model. The objective is to identify underlying structures and patterns in the data. This method is particularly beneficial for anomaly detection or clustering tasks, where the model must recognize data patterns without prior knowledge.
3. Reinforcement Learning
Machine learning utilizes Reinforcement Learning, a technique that teaches a model to make decisions based on rewards that incentivize positive behavior while discouraging negative behavior.
Reinforcement learning is usually implemented for tasks such as game-playing or robotics. The model must learn and adjust through trial and error to fine-tune its output and maximize performance.
Real-World Applications of Machine Learning
Machine learning has proven transformative in numerous industries, including healthcare, finance, retail, manufacturing, and social media. Machine learning has enabled businesses to make more informed and efficient decisions by automating decision-making and identifying data patterns, thus saving valuable time, money, and resources.
1. Healthcare
Machine learning has been a valuable tool in healthcare, revolutionizing disease diagnosis, drug discovery, and personalized medicine. For example, with machine learning models, medical images can be thoroughly analyzed to detect even minor abnormalities and provide pinpoint results that doctors might miss.
Predicting patient outcomes and identifying high-risk patients can be done accurately, helping doctors provide more effective and targeted treatments.
2. Finance
The realm of finance has been revolutionized by machine learning, which has given rise to automated systems designed to optimize investment strategies, detect fraudulent activities, and predict credit risks.
These systems exploit machine learning models to analyze immense amounts of financial data, detecting anomalies and patterns indicative of financial crimes, such as credit card fraud. They can also identify potential investment opportunities by studying market trends and historical data while predicting whether borrowers may default on their loans.
3. Retail
Machine learning has made its mark on the retail industry, where it is now used to personalize recommendations, optimize pricing strategies, and improve supply chain management.
Using customer data, machine learning models analyze patterns and preferences to enable retailers to make targeted customer recommendations or promotions. Retailers also use machine learning to optimize prices with insights obtained from market demand or inventory levels while predicting demand and optimizing inventory levels.
4. Manufacturing
Machine learning has changed the manufacturing industry, where it is now primarily used to optimize production processes, minimize downtime, and enhance quality control.
Manufacturing companies use machine learning models to evaluate sensor data from production equipment to identify potential inefficiencies, and real-time adjustments are made by manufacturers. Machine learning is also being used to forecast equipment failures and maintenance needs, which has resulted in reduced downtime and improved efficiency.
5. Social Media
Social media platforms utilize machine learning to customize content, improve visibility, detect false news, and enhance ad targeting. In addition, machine learning models on social media can scrutinize user data to identify their preferences and interests, making it easier to offer personalized content recommendations.
Another fundamental use of machine learning is detecting fake news or false information shared on social media sites. Social media platforms use machine learning to gather user demographics and behavior for better ad targeting.
Challenges in Machine Learning
While the potential benefits of machine learning are vast, several challenges and limitations must be addressed to realize its potential fully. Some of the key challenges in machine learning include:
1. Data Quality
The data quality used to train machine learning models is crucial for their accuracy and reliability. If the data used to train a model is biased, incomplete, or inconsistent, the model will likely make incorrect or unreliable predictions. Therefore, ensuring that data is of high quality and free from bias is crucial for the success of machine learning applications.
2. Interpretability
Machine learning models can be highly complex and challenging to interpret, making it tough to understand how they arrived at a particular prediction or decision. This lack of interpretability can make it difficult to trust or use machine learning models in certain contexts, such as healthcare, where decisions can have significant consequences.
3. Ethics and Privacy
The decisions made by machine learning models can have significant ethical and social consequences, such as influencing an individual's access to healthcare or employment opportunities. Hence, ensuring that machine learning applications are fair and ethical is paramount. Furthermore, machine learning models tend to depend on sensitive personal data, instigating apprehension regarding privacy and security.
4. Technical Limitations
While machine learning has advanced rapidly in recent years, technical limitations must be overcome. For example, machine learning models often require large amounts of computing power and memory, making them difficult to deploy in resource-constrained environments.
Additionally, some machine learning algorithms may be better suited to certain types of data or applications than others, requiring careful consideration and selection.
Future of Machine Learning
As machine learning continues to advance and make strides, its future continues to appear promising. Several trends and advancements in machine learning are anticipated to shape its future:
Advances in deep learning
Deep learning, a subset of machine learning, entails training neural networks with multiple layers. This technique has already paved the way for breakthroughs in applications like natural language processing and image recognition and is expected to progress further.
Increased Use of Reinforcement Learning
Reinforcement learning, which involves training models to make decisions based on a reward system, is becoming increasingly popular in robotics and game-playing applications. As more data becomes available and reinforcement learning techniques continue to advance, this type of learning is likely to become even more widespread.
Emphasis on Interpretability
The increasing complexity and widespread use of machine learning models imply an amplified requirement for interpretability—understanding how a model reaches decisions or predictions. This is expected to propel the development of techniques to make machine learning models more transparent and interpretable.
Growing Use of Transfer Learning
The transfer learning method comprises training a model on a specific task and eventually transferring that knowledge regarding a related endeavor. This approach is handy when data is scarce or expensive. This method is expected to become more widely adopted with increased data availability and advancements in transfer learning techniques.
Increased Focus on Ethical and Responsible Use
As the usage of machine learning becomes more widespread, there is a growing acknowledgment of the importance of ensuring ethical and responsible practices surrounding it.
Such practices include preventing biased data and discrimination against particular groups through models. Therefore, it is anticipated that there will be a heightened emphasis on developing ethical frameworks and best practices for using machine learning.
Take the Next Step in Machine Learning Today
Machine learning may seem complex initially, but as showcased in this beginner's guide, it need not be intimidating. With consistent practice and perseverance, anyone can grasp the basics and commence building their own models.
From forecasting customer conduct in a commercial setting to helping with medical diagnoses, the prospects of machine learning are infinite. Therefore, don't hold back—allow your imagination to run free, and start your machine learning journey today!